“Amaze Your Customers With Spectacular Findings from Survey Data.”
How Consultants Turn Their Customers Into a Life-Long
Cash Source Using Suprising Insights e.g. How to Boost Brand
Perception, Customer Loyalty or Sales Effectiveness.
By Frank Buckler, PhD -
Printable version
Why you have to read this article?
How would it boost your business if you extend your services with another highly differentiated field, overnight? How would you be able to amaze your customers, if you drew mind-blowing cause-effects insight from survey data, which where not possible until now?
As you read every word of this article, you will learn why existing analysis methods are of limited use,
why nobody until now developed a solution, which exiting oportunities
arise thru a potential new methodology and most importantly how you
will be able to profit from it tomorrow.
Why are existing analysis methods of limited help?
Issue one - spurious correlation:
Most of the time results of surveys are presented
like: "Successful enterprises invest on average 20% more into research
& development". From this one concludes that it will foster your
success to invest more into R&D. In fact, the chances are significant
that this conclusion is wrong. The
reason are spurious correlations. Maybe it is e.g. the innovative
company culture which spreads its positive influence in every
department and as a side effect makes the top management more willing
to spend an extra into R&D. And maybe the R&D department itself
causes more costs then effects, but get overcompensated by the
productivity of the employees working culture.
Because spurious correlations, nobody should
draw causal conclusions -when ever possible- out of descriptive or bi-variate analysis. You
always have to take all relevant data within one analysis into account.
Even though 95% of all analysis and conclusions drawn in day-to-day
business are descriptive or bi-variate, this doubtlessly remains a severe mistake.
Spurious correlations can only be avoided using Multivariate Analysis as
multiple regression. When data of all relevant terms are present, this
methods calculate the direct effect of one term on the other.
Issue two - latent variables and interconnectedness:
When it is about human, one wants to control things
like customer satisfaction, loyalty or attitude towards a brand. To
reliably model such terms you have to measure them in several, unique
ways, because this term itself can not be observed directly. The
results are combined into indices or latent variables. Only after this
step one is able to analyse the causes of this terms. Standard
multivariate analysis methods are not designed for this two-step
process.
Furthermore causes often influence each other and are not
independent to each other - this contradicts the working assumption of
multivariate methods such as multiple regression.
To solve all this issues, methods of Structural Equation Modeling were
developed. Until today there is little use of this methods in business applications. The main reason lay behind the
following causes:
Issue three - Unknown relations and properties:
In order to conduct Structural
Equation Modeling one has to know in advance, which term is influenced
by whom. In practice the detailed knowledge of all interrelations is
rather rare. Moreover the requirement to your data are quite
high as they often have to scale metrically and should be of
Gaussian distribution. All this are knock-out criteria for business
applications.
Furthermore Structural Equation Models are restricted to linear
relations and doesn’t allow interactions between causes. In fact such kind of
relations can be found quite often in reality. Bad enough that
existing methods forbid to model real life relations, the most severe
limitation is that: if you can not describe in detail all existing
relations prior analysis, todays methods will not help you to find out.
As a consequence, most Structural Equation Modeling studies are “wrong”! Professor Hennig-Thurau and I took a deeper look into four arbitrarily
chosen datasets, published in the world’s most reputed scientific
journals “Journal of Marketing Research” and “Journal of Marketing”.
We found in ever study clear indications for other unknown relations,
for nonlinear effects or interactions. If worlds leading researcher
fail to sensibly exploit today’s methods, how
should ever practitioner do so?
If problems are so obvious, why did nobody developed a solution
The short answer is: The solution is not obvious.
The detailed answer lies in the following four reasons:
- First of all, the mathematical paradigms of today’s methods (Structural Equation Modeling) are not suited to solve exploratory problems. Because of this it is
hard to think of an improved Structural Equation Modeling method that
solve the problem.
- Furthermore, the Structural Equation
Modeling research community is dominated by a confirmatory research
approach and often simply does not belief in a solution to this obvious
problem.
- Modern multivariate and exploratory methods as Artificial Neural Networks experienced major developments just in recent years.
- Latest methods such as Artificial Neural Networks were not suited since they suffer from the Black Box Problem: they
increase predictive performance but fail in conveying the
“why”.
Imagine a solution ...
... that is able to explore cause-effect-relations
with little a priori knowledge… that is able to reveal u-shape
relations if existent… that shows you that improving sales only
works if you deploy direct marketing and radio advertising jointly.
Case study: Brand image analysis
A regional utility provider asked its consultant to
figure out: “How does a green image improve customer
loyalty?”. We took the customer satisfaction monitor surveyed
every year and searched for relations between customer loyalty and the
image components. As a result we presented this:
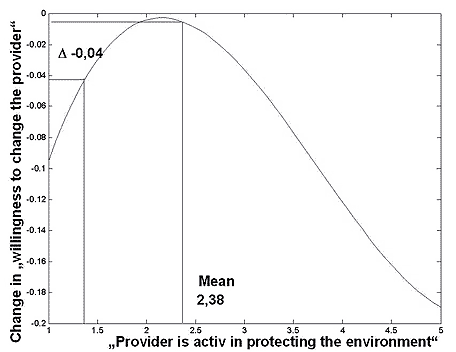
Here is what we told the customer about the graph:
“Dear Mr. Customer, a moderately green image is perfect and your
image is today already close to perfection. By focusing on green
initiatives
you might win some ‘tree hugger’. All other customers get
the impression that you would waste money instead of lowering
prices.” The customer was impressed – he boiled down the
wrong-leaded image campaign and saved 2 million..
Case study: Marketing-Mix Optimization
A woman cloth retail store chain wants
to boost profits and ask his consultant for help. When analysing a
customer survey data set we found out that perceived relationship
investment is a main prerequisite for repetitive purchases.
With our
analysis we showed…

… that excellent interpersonal communication
with customers is doing all the work. Expensive “tangible
rewards” (especially free gifts as shoe polish) are only an
alternative but a less effective tool. Just by skipping that, we cut
1,5% of overall costs, which boosted the profit by almost 30%.
Case study: Customer Loyalty Analysis
A national cellular network corporation asked its consultancy to find the specific driver of customer loyalty. Our analysis revealed the following graph:
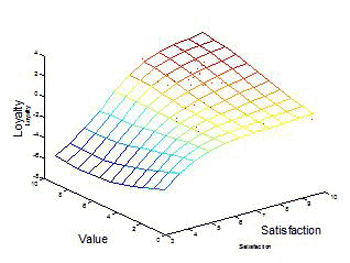
Using this, the customer learned the following lessons:
- Medium satisfaction is sufficient to keep customer loyal
- Unlike textbook theories, “value for money” has a direct causal influence on loyalty
- High perceived “value for money” increase loyalty only if a customer is already satisfied
With this we helped our customer to craft a very efficient
strategy that focuses on eliminating “satisfaction killer”
instead of “getting perfect”. Complain-response process got
optimized. Instead skipping some discounts for unsatisfied customers
save the company 1,5 million.
Which methodology can do this all?
The answer is "Universal Structural Modeling". The
foundation for USM was laid out in a five-year research project
conducted in cooperation with Harun Gebhardt - a project in which we developed a
stock forecasting system based on Neural Networks. In 1999 we launched
Profit-Station.com. The proven hit rates can still be experienced today
on a daily basis. In the same year I started my doctorial studies with
the ambitious goal to reinvent Structural Equation Modeling - the crown
jewels of social sciences. In 2001 I published the book "NEUSREL" which
introduced a new causal analysis method based on the same Neural
Networks that have already made Profit-Station successful. In successive
years the method was applied and refined in research and consulting
projects. Furthermore it matured in elaborate scientific discussions
with globally leading researchers. Important improvements were
stimulated thru Professor Hennig-Thurau. As a result the methodological
group "Universal Structural Modeling" (USM) for NEUSREL was
formed.
How does USM work? Causal-effect networks are built in two steps:
- The measurement level, where survey data get compressed to latent variables
- The structural level, where causal-effect relation between latent variables are analysed
At the measurement level I use principal component
analyses to compute the latent variables. At structural level a
specific Neural Network is trained for every dependent latent variable,
determining the influence of all latent variables. The type of Neural
Network used ensues that irrelevant effect path’ are killed. The
black box problem is mainly tackled by a methodology introduced by
Plate in 1998. It allows visualizing the separate causal effects.
That’s it.
If you like to know more, the best way is to consult my latest
scientific article published in “Marketing – Journal of Research and Management” which I co-authored with Professor Hennig-Thurau.
Interested readers I give the opportunity to get this article for free as a PDF per Email. Send an Email with your Name, Phone, Position and Organisation to
usm( at )neusrel.com.
How you profit from USM?
A lot of readers asked me how they could profit from
USM in their business. In order to enable a quick and cheap start,
first-time users can use my analysis service.
You fill an Excel-template with data and option settings – and I
run the calculations and send you the results per Email. Take advantage
of it to experience USM by yourself. For frequent users of USM I
provide a software licence.
Here some reference users that already used USM:
- Leading market researcher and consultants as GFK, B2Con Unternehmensberatung, Brandezza AG, Whiteboxx, CFI Group - Claes Fornell International, Burke Inc., InfoSearch, ...
- A growing number of innovative companies e.g. T-Mobile, Greif Inc., Sal. Oppenheim, Abbott, Procter & Gamble, L'Oréal, ...
Quotes from Users:
„We are conviced about NEUSRELs capabilities“
Mag. DI Ryffel GFK Trustmark
"... congratulations on creating a wonderful product--I am going to be
recommending it at places that I already have connections with."
John Steele, M. S., ABD, Kansas State University & Army Research Institute (ARI)
"... thank NEUSREL we were able to uncover important nonlinearities within a psychologic brand impact model."
Gregor Waller, lic.phil. Scientific Director, Brandezza AG
"The program provides very interesting diagnostics which give me a lot
of clues to dive into more insightful investigation of the data.".
Jae Cha , Chief Research Scientist , CFI Claes Fornell International
"I have applied the NEUSREL software designed by Dr. Buckler to
customer satisfaction and loyalty data, and found that it provides some
very desirable features. I have been happy about its ease of use,
functionality, and new and desirable features such as the ability to
identify non-linear and interaction effects in the model.”
Kunal Gupta, Ph.D. Vice President, Burke, Inc
We used Neusrel for exploring product adoption drivers. Thanks to
Neusrel's capability to include all kinds of variables (e.g. moderators
or categorial variables as gender) into our analysis, we were finally
able to avoid spurious findings and to derive some meaningful
recommendations concerning our proposition design and go-to-market
strategy.
Daniel Klein, Senior Manager, T-Mobile
What experts say about USM:
-
“I had the chance to read the book NEUSREL in 2001 as an early draft. Within
the scientific tradition of data-mining, I believe that both NEUSREL
and Universal Structure Modeling (USM) add a powerful instrument to
uncover hidden, more complex, and perhaps meaningful relationships
among variables."
Prof. Dr. Dr. Rene Weber, University of California at Santa Barbara, USA
-
“I use USM whenever I am working on a problem that
falls within its capabilities, for example, to estimate structural
equation models with many nominal variables such as gender. In the
field of customer confusion we found that confusion is particularly
prevalent among medium-income consumers, whereas low- and high-income
consumers employ buying heuristics that shield them from confusion. A
simple finding, however one we would have never found without
USM”,
Professor Dr. Gianfranco Walsh, Strathclyde Business School, University Glasgow & University of Koblenz
- “We are planning to apply USM for communication
controlling and planning in the advertising-intensive food industry. We
estimate to save companies a considerable part of their communication
spendings”,
Professor Dr. Holger Buxel, University of Applied Science Muenster
- In
contrast to classical methods of linear structural modeling NEUSREL
offers three advantages: Exploration capabilities, nonlinear relations
and arbitrary interactions between constructs are allowed and
will be considered. Due to this causal relationship structures tends to
get more realistic. Only if the data perfectly match all quite
restrictive assumptions of classical covariance-based methods results
might get possibly better.
Prof. Dr. Volker Trommsdorff, Technical University Berlin
- USM
allows an exploratory modeling of structural equation models. With this
quasi-confirmatory method new pathes, unknown nonlinearities and
interactions can be discovered, described and quantified.
Professor Dr. Rolf Weiber, University Trier
- “With NEUSREL Dr Buckler introduces an outstanding
contribution to marketing research, that has the potential to close a
major research gap"
Professor Dr. Klaus-Peter Wiedmann, University of Hanover
- "Best wishes as you expand the influence of this exciting software", Christopher P. Blocker, Ph.D. Assistant Professor, Hankamer School of Business, Baylor University
- "[The inventor of PLS] Wold talked about a dialog between the researcher and the data,
facilitated by the method. ... I think a tool like NEUSREL brings
PLS closer to Wold's original intent for PLS.".
Edward E. Rigdon, Professor, Department of Marketing, Georgia State University
-
“I
very much enjoyed the MJRM article about NEUSREL and I am particularly
intrigued by the non-linear/interaction capabilities.” Professor Dr. Claes Fornell, University of Michigan
How to summarize all said?
Today's analysis methods are design to test
existing theories and are not designed to explore new paths, unknown
nonlinearities and moderating effects. But exactly this is needed to be
useful in practical applications.
A solution to this problem was not developed so far
since scientific community did mainly ignored the practical issue.
Furthermore it was necessery to pursue a methodically new approach. The
foundation to this new approach where just developed in the last
years. Thats why a method as USM where only possible since then.
USM (Universal Structural Modeling) is a new causal
analysis using artificial neural networks, which plays for the
following advantages ...
- Exploration: Less a priori knowledge needed
- Nonlinearity: Explores (even unknown) nonlinear relationships
- Interactions: Finds, shows and quantifies interactions between causes
- Universality: Makes use of arbitrary
distributed variables. Especially nominal scaled variable as gender,
profession, brand name, etc. And: it is able to model circular causal
networks – no need to distinguish between endogen and exogenous
variables.
- Quantification: No matter if for
path strength, linear path coefficient, interaction strength or
significance figures, every important property get quantified.
- Simplicity – Easy to use, no need for detailed option settings.
Clearly, numerous success stories show the huge value
USM delivers. In nearly every sizable corporation the deployment of USM
can save millions in costs and foster for millions in additional
profits.
With the aid of my analysis service and a test of a
software licence, you have the chance to experience the potential of
USM on your own data. This is your step towards amazed loyal customers,
which will lead to significant additional profits.
Contact me and we will evaluate together the value USM will deliver to you.
Frank Buckler
Email: Buckler( at )neusrel.de